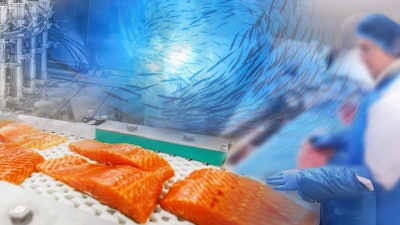
Salmon processing is big business, tapping into technology to stay innovative and globally competitive. While artificial vision algorithms can be applied to enable highly precise object detection – evaluating and counting fish in real time – they require specialized hardware that can consolidate workloads effectively. This goal resembles a data center priority, but in a much more unstable and physically rigorous environment.
It creates an engineering challenge that puts a spotlight on automation roadblocks common to the production or manufacturing floor - how to facilitate AI and machine learning in industrial environments that are nowhere near as precisely controlled as a clean, dry building full of servers.
Automation upgrades are increasingly strategic objectives among heavy industrial operations, even as engineers are often tasked with designing compute solutions for highly unique, IoT-based scenarios where they lack expertise. Standards-based rugged edge inference computers are breaking new ground in this effort, engineered for algorithm and workload performance that drives autonomy with data, localized processing, and the neural network capabilities necessary to accommodate the pace and massive scale of advanced automation.
Freshness Counts - For Fish and Data
More data calls for smarter data management and access, two factors that are essential in establishing greater levels of automation. The fish processing example relies on a large bank of data gathered and shared in real-time by smart sensors.
For instance, fish must be quantified and qualified as they come directly off the boat, with production line decisions that could be handled manually by a human workforce. Crucial to pricing levels and distribution plans, these choices rely on real-time assessments of size, color, and clarity. A challenging task at best, made even more difficult by improvements in commercial fishing operations that means more fish entering the production line at any given moment.
Human labor may create incorrect counts as well as quality control issues based on more subjective product evaluations, and there is obvious difficulty in maintaining pace with fish processing machinery handling as many as 100,000 fish per day. Alternatively, these same quantity and quality control operations can be well-managed by rugged edge inference computers coupled with software algorithms running processes in real-time.
Capitalizing on inference capabilities, or the creation of new logic from old logic, algorithms can steadily become more proficient at counting and appraising the quality of fish fillets with incredible speed and accuracy.
Neural Network Training
The incoming flux of data trains and retrains the system’s neural network and the rugged edge platform operates as a data aggregation device for this purpose. All visual data is persistently fed into the system, helping to perfect knowledge used for object detection. Once the neural network learns how best to recognize fish, the algorithm is deployed for use in rugged edge computers on the production floor.
In this scenario, a fish is delivered quickly after it is caught, entering the production line straight from the fishing vessel. The environmental considerations of this computing environment are extreme – and the rugged edge computer itself is validated to handle shock, vibration, severe temperatures and corrosive salt water.
Exceptionally robust design of rugged edge systems considers both physical design and compute performance, purpose-built to eliminate downtime and ensure stable 24/7 operation. Systems are ruggedized via mechanical and thermal engineering, constructed to assure performance in operations featuring heavy vibration, harsh temperatures, and elements of moisture and dirt. These industrial-grade computers are also performance validated, executing functions based on extreme processing power and storage capacity.
Along with programmed sensors and customized software algorithms, fanless rugged edge computers incorporate a spectrum of multicore processor options. These commonly blend CPU, GPU, and VPU compute elements to optimize systems’ ability to analyze images at high speeds – adding critical value and speed to this task, beyond human capacity in real-time.
Data movement is supported by 1Gbe connectivity, along with wireless data communication options such as Bluetooth, Wi-Fi, and cellular 4G LTE technologies. Coupled with faster storage drives, these performance accelerators allow real-time local processing without reliance on cloud processing, eliminating data bottlenecks that can result from massive amounts of real-time data.
Data processing is performed at or near its source, creating favorable impact on latency, privacy and security, and bandwidth. The results are significant improvements in productivity and efficiency gains, based on gleaning compelling data that significantly improves strategic decision making.
A Tackle Box for Food Manufacturers
Today, a blend of AI, IoT, and connectivity work in concert to help processing plants meet production goals, increase factory efficiency, and improve overall safety. Machines and data must closely partner, even as applications struggle with the physical demands of their environment and are highly sensitive to latency, bandwidth, and reliability issues.
Smart hardware strategies are playing a greater role on this landscape, enabling the software performance that allows heavy industrial operations a new level of access to real-time decision making. Powered by fast, predictive analytics at or near the data source, industrial operations are tapping into machine learning and inference requirements at the edge – driving more advanced automation capabilities, no matter the physical rigors of the end-use environment.
Premio is a global solutions provider specializing in computing technology from the edge to the cloud.