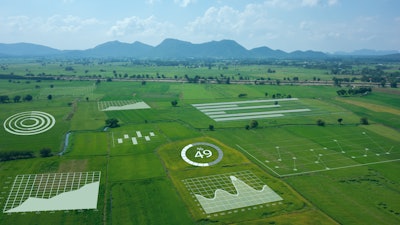
Food manufacturers well know that agribusiness is a tough business. The fact that people do tend to eat and drink is of some comfort. But competition is fierce, climate and environmental risks are growing, commodity markets are fickle, trade tensions are real, consumer tastes can pivot on a dime, and regulators are more and more demanding.
Artificial intelligence can’t solve all these problems, but AI-embedded software interacting with the intelligent agriculture solutions of enterprise resource processing vendors is tackling many of them.
Emerging AI applications for agribusiness
To pick out a few examples of AI solutions in action: Agranimo combines soil sensor, weather, and satellite data to maximize the yields of fresh produce that, separately, Clarifruit applies machine vision to quality-inspect. Solena’s AI determines soil health and quality based on microbiome, climate, crop, and soil features. And Vista brings together satellite, topography, soil, weather, hydrology, crop-physiology, and other data to minimize inputs, maximize yields, and provide the visibility to make better business decisions.
Those companies aren’t alone in deploying AI in the service of agriculture. AI chatbots in India are providing farmers with site-specific advice to address challenges such as droughts and pest infestations. The global seed supplier KWS uses AI to optimize seed genetics to help farmers grow resilient crops tailored to local conditions. AI can help evaluate suppliers, analyze transportation routes to minimize emission and reduce spoilage risk during transit, and scenario-plan for potential supply chain disruptions from severe weather to geopolitical unrest.
Early AI adopters such as Royal Eswatini Sugar are seeing payoffs. The South African sugarcane producer’s use of AI to analyze agronomic, satellite, and weather-modeling data boosted yields by roughly $5 million, saved $157,000 in costs per season, and brought a 10% increase in forecast accuracy.
AI for agricultural compliance
There’s another area of emerging agribusiness AI worth noting. It’s addressing an increasingly pressing problem: traceability and compliance in an environment of complex and sometimes conflicting compliance mandates. The U.S. Food Safety and Modernization Act’s (FSMA) section 204 deadline is months away – Jan. 1, 2026. Two days before that, the recently delayed EU Regulation of Deforestation-free Products (EUDR) will go into force. Layer in the recently passed EU geographical indication schemes, local regulations, and the policies of major customers, and it’s clear that visibility into the precise provenance and pathways of agricultural products is no longer optional.
Consider the example of a major producer of fresh fruit. They must adhere to a mix of global regulations and standards, industry standards, and local rules in every country in which they produce. Those rules may differ in intent even if they align linguistically. For example, the need to ensure availability of “sanitary installations” at agricultural production sites may mean four walls and a roof in one jurisdiction and a fully climate-controlled space in another. Then there are the compliance mandates where the fresh fruit is sold and the varying standards of major buyers.
Generative AI is built for compliance work
In the past, meeting the challenge of compliance-requirements analysis has involved people poring over and reconciling thousands of pages of documents, establishing the policies to follow at various junctures, and nailing down the exact data points needed to prove and document compliance. In many cases, this data also comes from upstream growers and business partners through surveys, audits, or automated capture during field and farm fertilization and crop-protection activities.
Once those rules are established, continuous tracking of regulations is crucial to ensure that compliance approaches adjust as rules change. This sort of effort plays into the strengths of Generative AI (GenAI), and msg global is among those exploiting it in the area of compliance.
The Germany-based firm has developed a GenAI tool that can help agribusinesses scrape relevant documents, be they municipal or international, and synthesizes them into clear statements about the specific compliance obligations a given agribusiness must follow, from the field to the buyer’s facility. As regulations change, the system automatically updates compliance obligations, propagates them across affected areas, and supports the validation and update of internal policies.
GenAI as interviewer and reporter
Of course, distilling requirements is only the start of a compliance effort. GenAI can also play a central role in maintaining compliance. A GenAI agribusiness compliance system can ask farmers, logistics experts, buyers and sellers, managers, or anyone else questions in plain English (or Spanish, or Swahili).
The answers help clarify compliance status and generate draft reports tailored to appropriate regulatory levels. Also worth noting is that the data collected for compliance purposes also serves as feedstock for other business AI systems to digest and exploit.
Agribusiness’s geographic dispersion and rural nature does bring challenges to AI deployment. They range from a lack of financial resources to limited internet connectivity to skills gaps. Overcoming them will take collaboration, government support, educational initiatives, and the development of cost-effective, scalable AI solutions that smaller operations can handle. But from precision agriculture to compliance, those investments will continue to pay off for agribusiness – and for its customers.
Cedrik Kern is an SAP solution manager for agribusiness and commodity management.
Eric Somitsch is SAP’s senior director of agribusiness and commodity management.