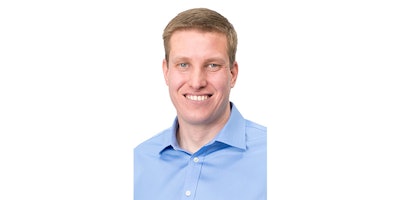
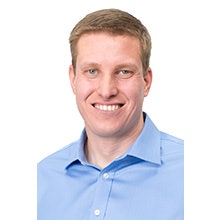
At one time, food and beverage manufacturers could only rely on paper and pencil for quality data collection. Plant staff spent their shifts furiously scribbling down measurements coming off production lines. Unfortunately, they could not capture every possible measurement that would lend itself to process control or quality improvement. But now, with the rise of automation, Big Data, and the Industrial Internet of Things (IIoT), it is easier than ever for food producers to collect comprehensive data about their production processes.
Whereas many manufacturers were once starved for data, a new challenge has emerged: they now have too much data on their hands. At the same time, these companies are managing increasingly complex supply chains—with multiple plants and suppliers located across regions and even around the world. The sheer volume of data coming from these sites can be overwhelming for any quality professional. Many are left unsure about how to sift through the noise of data to uncover actionable intelligence that can benefit individual plants or the entire enterprise.
Below are three steps for food and beverage manufacturers to follow to find clarity in the noise, identify areas that require attention, and prioritize process improvements.
1. Centralize All Quality Data In One Place
More often than not, food producers with multiple plants and suppliers suffer from data silos, as each site uses its own databases, storage systems, and naming conventions for data collection. The disparities, physical separation, and lack of standardization make it difficult to perform any sort of cross-plant analysis. Using the cloud, manufacturers can instead store their quality data in one unified data repository.
With data in one location, manufacturers break down existing silos and promote standardization across all plants. The result is enterprise visibility, which gives quality professionals insight into not only the quality metrics within individual locations, but also production performance from site to site and across all regions. The big-picture view of quality they gain enables them to begin to explore global improvement initiatives.
2. Prioritize Quality Efforts And Resources
Using the cloud and new quality intelligence tools, food and beverage manufacturers can aggregate and roll up the massive amount of data from across their organization to the corporate level for analysis. From here, executives and decision makers can extract valuable operational insights that will help them to improve performance at the site level and enterprise wide. Using these insights, companies can easily identify where the greatest opportunities for improvement lie—and prioritize quality efforts and available resources accordingly.
For example, a quality issue may simply require a small change on some equipment settings. These are “easy wins” (i.e., they require little effort but can deliver big impact on operations). Some projects may require higher-level expertise or greater expenditures, such as completely replacing a piece of equipment or upgrading an entire filling line. Knowing the root cause of an issue and the effort required to fix it enable manufacturers to effectively plan process improvement budgets based on return on investment.
3. See What Makes The Grade
The third step can be considered part of the second. Known as “grading,” it is an innovative new way to cut through data noise. Using the vast amount of aggregated data in a unified centralized data repository, grading as a function automatically applies Statistical Process Control (SPC) techniques and analysis to generate a simple letter-number combination that represents both expected and potential yield for each product, line or process—thought of as “streams.” Grades can also represent entire production sites.
A rank of A, B, or C indicates each stream’s yield potential. A rank of 1, 2, or 3 can be used to rank the same stream’s yield performance. These letter-number combinations inform executives and quality teams, at a glance, how each aspect of manufacturing is performing—and if it is living up to its full potential. For instance, an A1 grade is a high-yield stream that is meeting potential. In contrast, a C3 grade is a low-yield stream that is not meeting potential.
Together, these yield metrics offer manufacturers a new way of looking at quality performance that enables rapid prioritization. Grading provides the ability to drill down, layer by layer, and identify the root cause of reduced yield, enabling more targeted solutions.
Conclusion
As the volume of quality data continues to grow and compound across today’s enterprises, food and beverage manufacturers will need a way to cut through the noise and make agile decisions. Starting with centralizing quality data in a unified repository, organizations can begin to unearth insights that improve quality, drive efficiency, cut costs, eliminate waste, and more. By applying new techniques in understanding performance, like grading, they can determine where and how to allocate resources for the greatest business impact—and become more competitive on a global scale.
Eric Weisbrod is Vice President of Product Management, InfinityQS International, Inc.