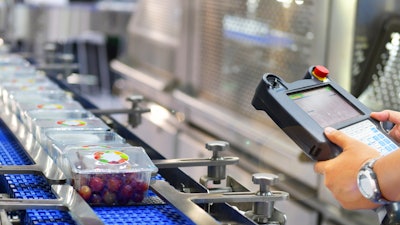
Up until March of this year, the food industry in the United States was remarkably stable, with consistent players, demand patterns, and revenue streams. But in April, everything changed: consumer demand volatility, workforce scarcity and density requirements and supply chain disruptions placed an unprecedented burden on manufacturers in the food industry.
These new challenges are compounded by the need to maintain the company’s image, reputation and brand, while maintaining the production line and producing quality products. Otherwise, manufacturers risk the potential for product defects which can lead to catastrophic consequences – such as expensive product recalls or possibly injuries, damaged equipment, legal ramifications, scrapped items, or lost customers – that ultimately impact their bottom line.
As manufacturers look to emerging technologies to help adjust to the “new normal,” there is a major opportunity for deep learning, artificial intelligence, and automation. In fact, a recent CapGemini report found that quality inspection is one of the most common applications for AI in the manufacturing industry, as it is enabling the adoption of automation that is faster, cheaper and more accurate than ever before.
Applying AI to visual inspections also provides greater flexibility in production line changes and shift agility, helping factories respond to the volatility in customer demand and preferences. Models can be created and deployed quickly to adjust to new product types, new components, or new packaging types, minimizing the engineering time for new inspection systems and the amount of line downtime required to implement new systems.
In food manufacturing, quality inspections occur throughout the production line. Whether it’s replacing human workers that were previously able to do the inspection or augmenting machine vision and bar code scanners, automating quality inspection with vision AI software can result in dramatic improvements. Here are several real-life use case examples:
Raw ingredients entering a factory
At a commercial bakery where large volumes of ingredients enter the factory daily, occasionally a supplier sends the wrong items. Historically this issue has been solved by adding a barcode reader. But sometimes the factory receives the wrong product with the right barcode, or even the right product with the wrong barcode, so a barcode reader alone is not accurate enough. There is a need to recognize the product visually and compare it to the scanned barcode, so that all possible errors are corrected before the ingredients are stored and used, improving the overall production of the bakery while minimizing waste.
After ingredients enter the facility, they are depalletized and placed on a conveyor belt with a bar code scanner. A vision AI enabled camera can be placed above a conveyor belt so that the barcode reader scans the barcode, taking pictures of the bags and boxes of ingredients while the AI confirms the type of product. If the barcode and expected product match, the ingredients continue on the conveyor; if they don’t match, the item is diverted off the line, and an operator is notified to either confirm that the correct package has arrived, just with a wrong barcode, or that the wrong item has been received and it needs to be returned.
iStock
Surface inspection of products on the line
To build on the bakery example, there are several other examples of where quality inspection occurs.
The first is before loaves of bread are baked, to ensure that their mold is filled properly and that the surface is correct (e.g. bubbles vs. no bubbles), so that the bread will bake properly. Similarly, as baked goods come out of a commercial oven, they are inspected for things such as color (to determine doneness), distribution of ingredients on top (chocolate chips, poppy seeds, sesame seeds, etc.) and shape outline.
Typically, inspections like these are done by hand, where a certain percentage of trays are pulled off the line. This requires a full-time employee and disrupts the manufacturing flow. With a different person on for each shift, consistency is challenging, and therefore rarely able to be maintained. To ensure quality, cameras equipped with vision AI software can be placed to view the baking trays before baking and after exiting the oven. The product can then be inspected at both intervals to confirm that the correct product is entering the oven, and that the bake was done successfully. As a result, more products can be inspected and defects can be detected earlier, minimizing waste.
Case packing
At the end of a food production line, case packing is frequently used for pet food, canned vegetables, canned soup, etc., either just for transportation (e.g. to a grocery store that then unpacks the cans and places them on a shelf), or to be sold as a unit to customers (like in a warehouse retailer that sells in bulk). Products are then stored in differently sized cans, packed in trays, then shrink wrapped before being sent to a palletizer.
In this instance, inspections are usually done manually by an operator, but only when there are problems at the palletizer. Each case is inspected very briefly to determine what is causing issues down the line – but this is too late. Since issues with packaging can cause issues at palletizing, there is a need to increase inspections at the case packing stage. Here, a vision AI-enabled camera can be placed after the shrink-wrapping station to watch the cases as they come out of the case packer. The result is a higher inspection rate without needing to increase the number of workers on the floor, all while preventing downtime at the palletizer due to improperly packed cases.
The final word
While it is possible that consumer demand and supply chain will one day return to pre-pandemic levels, food manufacturers need to act now and plan for a scenario where AI acts as an indispensable workforce multiplier to cope with these increasingly challenging times.
And perhaps we could look at 2020 as the year where AI-powered productivity gains helped truly materialize Industry 4.0.